
SIR Models
Equation 
The SIR type models consider three types of populations, the susceptible
As in
• the infection is not fatal,
• the model does not include birth
• the model does not include death from another cause
The total number of the population will be equal to the sum of the three groups:
$N=S+I+R$ |
ID:(871, 0)

Mechanism of contagion
Equation 
Probability that a person I find is infected is\\n\\n
$\displaystyle\frac{I}{N}$
\\n\\nthat is favorable cases
$C\displaystyle\frac{I}{N}$
\\n\\nOpportunities to infect a healthy. The probability of contagion will depend on the time you have contact. Suppose we consider a time
$\beta dt$
\\n\\nWith this in time
$C\displaystyle\frac{I}{N}\beta$
\\n\\nTo obtain the total number of infected in time
$C\displaystyle\frac{I}{N}S\beta$
that is
$i(t)=C\displaystyle\frac{I}{N}S\beta$ |
ID:(8106, 0)

Contagion mechanism - equation Susceptible SIR
Equation 
As the number of susceptible
$\displaystyle\frac{dS}{dt}=-i(t)$
you have to with
$i(t)=C\displaystyle\frac{I}{N}S\beta$ |
the equation to calculate the development of the susceptible population is
$\displaystyle\frac{dS}{dt}=-C\displaystyle\frac{I}{N}S\beta$ |
ID:(4068, 0)

Number of those who remain infected
Equation 
If for the
$\displaystyle\int_0^tdt'i(t')$
If we want to estimate those that still remain infected, we must subtract all that have been recovered to date, those that are
$I(t)=\displaystyle\int_0^tdtau i(tau)-R(t)$ |
ID:(3888, 0)

Variation in the number of infected
Equation 
To know the variation in time of the number of infected can be derived in time the equation
$I(t)=\displaystyle\int_0^tdtau i(tau)-R(t)$ |
with what you get the equation
$\displaystyle\frac{dI}{dt}=i(t)-\displaystyle\frac{dR}{dt}$ |
ID:(4069, 0)

Mechanism of recovery/death
Equation 
The question now is how infected people
$r(t)=\displaystyle\int_0^t dtau p(t-tau)i(tau)$ |
ID:(3889, 0)

Total recovered, SIR model
Equation 
If the number that is retrieved between the
$r(t)=\displaystyle\int_0^t dtau p(t-tau)i(tau)$ |
then the total recovered at the time
$R(t)=\displaystyle\int_0^t dtau r(tau)$ |
ID:(3890, 0)

Recovered Differential Equation
Equation 
As the number of recovered is
$R(t)=\displaystyle\int_0^t dtau r(tau)$ |
and the daily number is
$r(t)=\displaystyle\int_0^t dtau p(t-tau)i(tau)$ |
the derivative of the number of recovered is equal to
$\displaystyle\frac{dR}{dt}=\int_0^t dtau p(t-tau)i(tau)$ |
ID:(3891, 0)

Simplified recovery model - equation recovered SIR
Equation 
The amount of daily recoveries was calculated by adding on all the historical
$r(t)=\displaystyle\int_0^t dtau p(t-tau)i(tau)$ |
which corresponded to the daily variation of the total recovered
$\displaystyle\frac{dR}{dt}=\int_0^t dtau p(t-tau)i(tau)$ |
As the number of infected daily is
$i(t)=C\displaystyle\frac{I}{N}S\beta$ |
\\n\\nit is necessary that the variation of recovered is of the form:\\n\\n
$\displaystyle\frac{dR}{dt}=\displaystyle\frac{\beta C}{N}\displaystyle\int_0^t dt' p(t-t')S(t')I(t')$
\\n\\nIf the infected are few compared to the susceptible and the probability function decays faster than the number of infected varies the integral can be replaced by a constant\\n\\n
$\displaystyle\frac{\beta C}{N}\displaystyle\int_0^t dt' p(t-t')S(t')I(t')\sim\left[\displaystyle\frac{\beta C}{N}\displaystyle\int_0^t dt' p(t-t')S(t')\right]I(t)\sim\gamma I(t)$
with what the recovery equation looks like
$\displaystyle\frac{dR}{dt}=\gamma I$ |
ID:(4072, 0)

Rewriting the second equation SIR
Equation 
If the third equation of the SIR model is replaced
$\displaystyle\frac{dR}{dt}=\gamma I$ |
in the second
$\displaystyle\frac{dI}{dt}=i(t)-\displaystyle\frac{dR}{dt}$ |
and using
$i(t)=C\displaystyle\frac{I}{N}S\beta$ |
an equation is obtained in which one can understand the situations in which the disease spreads or is managed to control
$\displaystyle\frac{dI}{dt}=\left(\displaystyle\frac{\beta C}{N}S-\gamma\right)I$ |
ID:(4073, 0)

Approach of Survivors without contagion, SIR model
Equation 
As we saw, there is a limit in which the disease itself controls its expansion simply by the lack of susceptible to being infected. The question is what size is the group that is saved from infection. If we divide the equation
$\displaystyle\frac{dI}{dt}=\left(\displaystyle\frac{\beta C}{N}S-\gamma\right)I$ |
by
$\displaystyle\frac{dS}{dt}=-C\displaystyle\frac{I}{N}S\beta$ |
\\n\\nan independent equation of time (or variable to temporal u) of the form can be obtained\\n\\n
$\displaystyle\frac{dI}{dS}=-1+\displaystyle\frac{\gamma}{\beta C}\displaystyle\frac{N}{S}$
\\n\\nIf this equation is integrated assuming initial conditions
$I - I_0 = (S_0 - S) + \displaystyle\frac{\gamma N}{\beta C}\ln\displaystyle\frac{S}{S_0}$
\\n\\nTo determine the number of survivors we are interested in the case in which the situation has already stabilized and we no longer have infected (
$(S_0-S)+\displaystyle\frac{\gamma N}{\beta C}\ln\displaystyle\frac{S}{S_0}=0$
This equation cannot be solved in an exact way, however if the logarithm is expanded by Taylor until the second order is achieved, the size of the population is not infected.
$S_{\infty}=S_0\left(3-2\displaystyle\displaystyle\frac{\beta C}{\gamma}\displaystyle\displaystyle\frac{S_0}{N}\right)$ |
ID:(4077, 0)

Stationary condition
Equation 
Initially it can be considered that
$\displaystyle\frac{dI}{dt}=\left(\displaystyle\frac{\beta C}{N}S-\gamma\right)I$ |
\\n\\nthe factor\\n\\n
$\displaystyle\frac{C\beta}{\gamma}\displaystyle\frac{S}{N}-1 > 0$
\\n\\nis positive. This occurs if the factor\\n\\n
$\displaystyle\frac{C\beta}{\gamma}\displaystyle\frac{S}{N}$
\\n\\nIt is greater than one. As initially the susceptible population
$\displaystyle\frac{C\beta}{\gamma} > 0$
On the other hand, over time, the disease itself will reduce the number of susceptible people causing it to self-control due to the reduction of new victims. These situations will occur if the number of susceptible reaches
$\displaystyle\frac{S_{crit}}{N}=\displaystyle\frac{\gamma}{C\beta}$ |
which corresponds to the situation in which the infected curve reaches its maximum. In other words, the number of critically susceptible is the number of susceptible that remain at the moment that the number of infected reaches its maximum.
The number of critically susceptible subjects shows that preventive vaccination helps control the disease by creating a situation in which the initial susceptible are such that the disease is self-controlled.
ID:(4075, 0)

Reproduction Factor SIR
Equation 
Being critical is the factor that compares the factors of contagion
$R_0=\displaystyle\frac{\beta C}{\gamma}$ |
As a reference, reproduction factors of the most typical diseases are listed:
Disease | Transmission | $R_0$ |
Measles | droplets in suspension | 12-18 |
Whooping cough | droplets in suspension | 12-17 |
Diphtheria | saliva | 6-7 |
Smallpox | droplets in suspension | 5-7 |
Polio | fecal-oral route | 5-7 |
Rubella | droplets in suspension | 5-7 |
Mumps | droplets in suspension | 4-7 |
HIV / AIDS | sexual contact | 2-5 |
SARS | droplets in suspension | 2-5 |
Influenza (1918 pandemic strain) | droplets in suspension | 2-3 |
Ebola | body fluids | 1.5-2.5 |
ID:(4074, 0)

Limit Containment
Equation 
Another application of the equation is to allow estimating the necessary measures to avoid the epidemic. In general, the susceptible population needs to be less than the critical value.
$\displaystyle\frac{S_{crit}}{N}=\displaystyle\frac{\gamma}{C\beta}$ |
\\n\\nThe reduction of
$S_{crit}=(1-q)S$
With the recovery factor
$R_0=\displaystyle\frac{\beta C}{\gamma}$ |
\\n\\nit has to\\n\\n
$q=1-\displaystyle\frac{1}{R_0}\displaystyle\frac{N}{S}$
If it is assumed that initially the susceptible are equal to the population (
$q=1-\displaystyle\frac{1}{R_0}$ |
which gives us an idea of the level of mass that the vaccination campaign should have.
As an example in the diseases considered, we must:
| Disease | Transmission | Vaccination |
|: --------- |: ---------- |: ---------: |
| Measles | droplets in suspension | 92-94% |
| Whooping cough | droplets in suspension | 92-94% |
| Diphtheria | saliva | 83-86% |
| Smallpox | droplets in suspension | 80-86% |
| Polio | fecal-oral route | 80-86% |
| Rubella | droplets in suspension | 80-86% |
| Mumps | droplets in suspension | 75-86% |
| HIV / AIDS | sexual contact | 50-80% |
| SARS | droplets in suspension | 50-80% |
| Influenza (1918 pandemic strain) | droplets in suspension | 50-67% |
| Ebola | body fluids | 34-60% |
ID:(4076, 0)

SIR model simulation
Html 
The model can solve numerically the equations for susceptible
$\displaystyle\frac{dS}{dt}=-C\displaystyle\frac{I}{N}S\beta$ |
$\displaystyle\frac{dI}{dt}=\left(\displaystyle\frac{\beta C}{N}S-\gamma\right)I$ |
$\displaystyle\frac{dR}{dt}=\gamma I$ |
where
ID:(3022, 0)
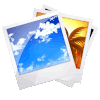
SIR model to describe SARS 2003 in Hong Kong
Image 
If the susceptible, infected and 'recovered' (who heal or die) are observed, the typical curves of the SIR model are observed:
ID:(9663, 0)