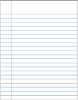
Routine zum Zeichnen von Matrix
Beschreibung 
def plot_confusion_matrix(cm,classes, normalize=False, title='Confusion matrix', cmap=plt.cm.Blues): plt.imshow(cm,interpolation='nearest',cmap=cmap) plt.title(title) plt.colorbar() tick_marks = np.arange(len(classes)) plt.xticks(tick_marks, classes, rotation=45) plt.yticks(tick_marks, classes) if(normalize): cm = cm.astype('float') / cm.sum(axis=1)[:,np.newaxis] print('Normalized confusion matrix') else: print('Confusion matrix, without normalization') print(cm) thresh = cm.max() / 2. for i, j in itertools.product(range(cm.shape[0]), range(cm.shape[1])): plt.text(j, i, cm[i,j], horizontalalignment='center', color='white' if cm[i,j] > thresh else 'black') plt.tight_layout() plt.ylabel('True label') plt.xlabel('Predict label')
ID:(13769, 0)
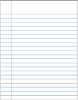
Datei zur Analyse generieren
Beschreibung 
# define directories work_directory = 'F:/go/face_scrapper/faces/work/' test_path = work_directory+'test/' # generate test batches test_batches = ImageDataGenerator(preprocessing_function=tf.keras.applications.vgg16.preprocess_input).flow_from_directory(directory=test_path, target_size=(224,224), classes=classes, batch_size=10, shuffle=False)
ID:(13770, 0)
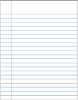
Datei mit Vorhersagen generieren
Beschreibung 
# erzeugen der Vorhersehung predictions = model.predict(x=test_batches, verbose=0)
ID:(13771, 0)
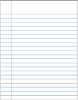
Datei mit Vorhersagen generieren
Beschreibung 
# calculate confusion matrix cm = confusion_matrix(y_true=test_batches.classes,y_pred=np.argmax(predictions,axis=-1))
ID:(13772, 0)
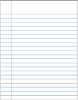
Verwirrungsmatrix anzeigen
Beschreibung 
# show confusion matrix cm_plot_labels = classes plot_confusion_matrix(cm=cm, classes=cm_plot_labels, title='Confusion Matrix')
ID:(13773, 0)
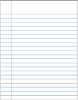
Verwechslungsmatrix-Statistik
Beschreibung 
import numpy # get trace n = numpy.trace(cm) # get sum s = numpy.matrix(cm) v = s.sum() # fraction of trace (correct) to all others ( f = 2*n/(v - n) # show result print(str(n)+','+str(v)+','+str(f))
ID:(13774, 0)